Alternative Data in Retail Sector

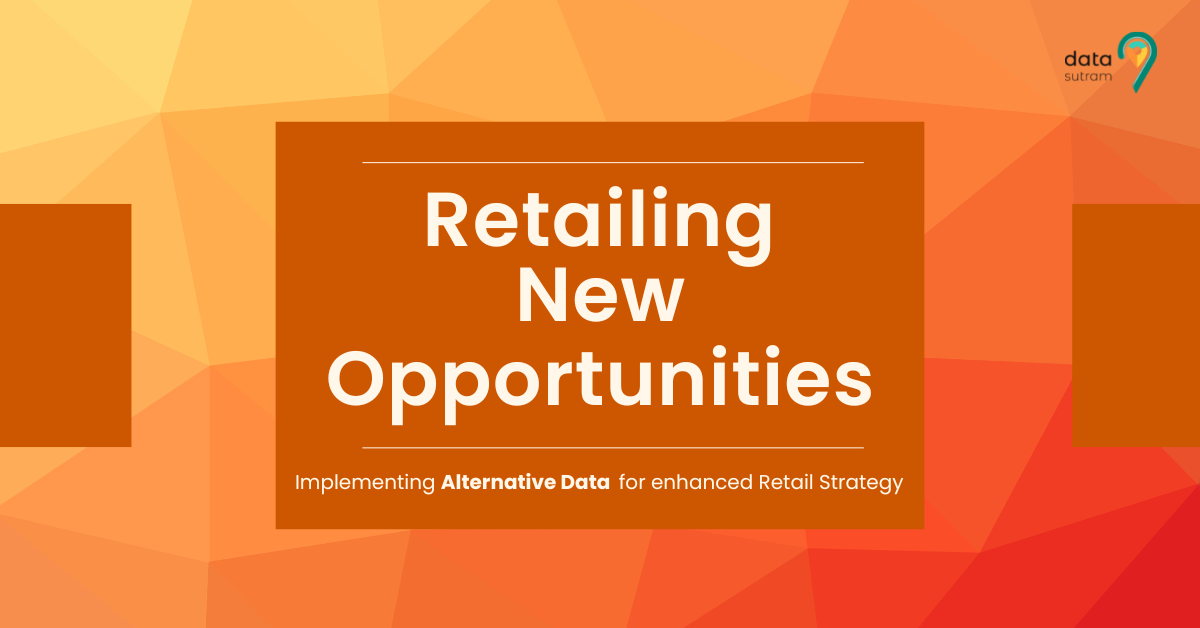
RetailSutra was one of the leading retail brands based out of Mumbai; just like its competitors, it was swamped while setting targets for the next quarter. The ambitious C-suite kept the hefty target of opening 100 new stores in the upcoming Q3 despite the hardships faced in the previous quarter.
RetailSutra was bewildered seeing the performance of the existing and newly opened stores in Q2. Despite extensive on-ground research, 90 of 100 stores faced infrastructural and inventory losses and weren’t profitable. The losses were so significant that even the ten most profitable stores couldn’t balance the other stores’ failures.
RetailSutra was an e-commerce and even owned Brick and Mortar stores with the idea of reaching out to every customer from any demography in the country. Given the nature of the product, the leadership could not compromise on the targets. However, there was still a hassle to devise an optimal plan of action to minimize losses and make good profits.
Meanwhile, Rahul Tiwari, who’s been part of RetailSutra for four years and delivering profits from his managerial region, was promoted and placed in the chakravyuh of opening the new 100 stores. Rahul was very aware of the losses faced by the company in the last quarter and had to plan every move smartly for an optimal plan. Rahul began analyzing what went wrong for the 90 loss-making stores.
He mapped out the 90 stores and segregated them based on the loss margin, demography, inventory costs, stock-keeping capacity and other factors to get a macro overview of these stores. He then looked at the pin codes where these stores exist and wanted to analyze the alternative parameters to profile these pin codes. During all of this, he came across Data Sutram. Knowing more about the company, he realized he could use their solutions to identify potential locations.
Initially, after mapping the loss-making pin codes, with the help of Data Sutram, Rahul was able to profile these locations based on parameters such as
- Affluence
- Footfall
- Income Groups
- Competitive Density
- Spending Behaviour
- Satellite Data
and 1k+ Data Sutram’s parameters. He went through the quantitative process to map micro market parameters to understand the socio-economic behavior using these parameters; Data Sutram helped Rahul with a location scorecard for all these pin codes. Using this scorecard, Rahul broadly divided the pin codes into two categories: profitable and non-profitable.
Rahul calculated losses at these locations and possible reasons concerning the above-identified parameters. He could devise a plan to optimize the existing stores to deliver profits and discard the non-performing stores, which simply can’t provide the ROI.
After an in-depth analysis of the past performance, Rahul could focus on the Q3 targets. The board was looking forward to opening stores in new locations across India, especially in Tier-1 cities and highly affluent pin codes. For new stores, Rahul took Data Sutram’s help, started profiling new sites, and got a macro and micro insight into the new demographics.
He looked at the current market penetration using Gap% and Store Saturation and conducted an opportunity analysis using DS parameters to identify the business potential of the sites. Rahul identified 50 ideal locations that would be profitable and resonate well with the company’s quarterly and annual targets. He conducted a working capital comparison of opening 50 stores at these ideal locations with opening 100 new stores at preferred locations. He realized that 50 out of the preferred 100 locations would face shortcomings and be affected by parameters such as whether it is a tourist place or the weather conditions. Subsequently, it’ll be non-profitable to open stores at these locations.
The company went ahead with Rahul and Data Sutram’s suggested locations and delivered profits with
- Optimizing the existing non-profitable stores
- Selecting highly potential sites for new locations.
Further, to maintain and drive these profits company was able to:
- Plan inventory for new & existing stores
- Hyperlocal Marketing
- Plan Resources & Hyperlocal Campaigns based on opportunity
- Accurate data-driven targeting
- Field-force management
- Reduce NPAs
And address several relevant use cases.
The key emphasis was to use such technology and parameters to study data before goal planning and target setting. Teams can use alternative data to optimize their course of action to reach their ideal goals without compromise.